When I first started talking about the Membership Economy, there just weren’t that many academics or researchers interested in studying the power of customer retention. That’s why I’m really happy to see some of the best minds in finance, strategy and marketing today are thinking about how to build, and measure durable relationships between organizations and the people they serve.
Today’s guest, Eva Ascarza, is the Jakurski Family Associate Professor of Business Administration at the Harvard Business School. Her primary research subject is customer management (with special attention to the problem of customer retention). That’s good news for all of us building subscription businesses. In today’s discussion, we talk about the right metrics to measure and improve customer retention, how to use pattern recognition to predict which customers will be most valuable, and why cohort-based analysis is so important.
—
Listen to the podcast here
Going Deep on Customer Retention Analytics for Subscriptions with Harvard Business School’s Eva Ascarza
When I first started talking about the membership economy, there weren’t that many academics and researchers interested in studying the power of customer retention. That’s why I am so happy to see some of the best minds in finance, marketing, and strategy, focusing on how to build and measure durable relationships between organizations and the people they serve.
Our guest, Eva Ascarza, is the Jakurski Family Associate Professor of Business Administration at the Harvard Business School. Her primary research subject is customer management with special attention to the problem of customer retention. That’s great news for all of us building subscription businesses. In this discussion, we talk about the right metrics to measure and improve customer retention, how to use pattern recognition to predict which customers will be most valuable, and why cohort-based analysis is so important.
—
Eva, welcome to the show.
Thank you very much for having me.
How did you come to become interested in customer retention as an area of study?
It was a bit random. I will fully admit it. I was never a marketer. I was never a marketing major. I did mathematics as an undergrad. When I finished, one thing led to another, and I got an internship at a bank as a CRM junior. I did not know that CRM meant Customer Relationship Management. I was told that I was going to be data analysis and they thought a person with a math background could do helpful stuff there.
I joined the bank. It was a very good opportunity because it was what I wanted to be location-wise. It was a good moment to have that job. In the job, I knew nothing about marketing. I helped these people with Excel analyzing data. It was a non-sophisticated bank back then. This was in 2001 to 2002. There was this day in which the CMO came to the marketing department running from the very senior board meeting where, all of a sudden, she was told that millions and millions of pesetas, which was the currency of Spain, were going to ING, the bank from the Netherlands who entered the market in Spain.
They were freaking out. They wouldn’t know what to do with that. As a CRM junior, all I did was extract all the money that have left the bank and summarize it. When they saw the quantity, they were amazed. What I was amazed about was that they didn’t know that the money had already left the bank. It’s not even to predict that people will be taking their wallets somewhere else. The money was already out of the bank.
For me, it was fascinating that a marketing department had not been looking at that. I did get an offer from the bank to stay. I didn’t take it and I went and do and did a PhD in marketing. It’s not that day I decided to do the PhD. There were other things that mattered at the time. The idea that all these customers, value, and money had left the bank and they didn’t even know was horrendous and fascinating at the same time. When I started the PhD, I knew I wanted to do something about how you figure out how people keep being your customers, and in the bank would be the money, but whatever service you have to them.
Money leaving the bank is about the most direct churn that you can ever measure because they literally take their money and put it somewhere else. I think about marketing often as being very focused on the front end of the funnel. It’s like once the transaction happens, that’s the finish line for me, and I go back out and try to find other customers. It sounds like what fascinated you is that you felt like they should have been thinking that more as the starting line for understanding the relationship.
Back then, I didn’t even know what customer acquisition was. It was not top of my mind the fact that they should be going out there and trying to figure out how to track more people and how to grow that way. It was clear to me that you have a relationship with the bank and the default would be to keep the money there.
The fact that all these customers actively went to the bank account to take the money out was so active churn. I didn’t maybe know the terminology back then, but the fact that was so active and the fact that they are taking their business elsewhere and they didn’t know was shocking and determining a little bit.
That was exactly the time when I started to become interested in retention as well. I was consulting with Netflix right around that time and was noticing the opposite, which was they were completely focused on who left when and why, which was so interesting because I’d been in product management. I’d never seen that before.
I’d never had a company that I’d worked for either as a big firm consultant or as a product manager that was as focused on what we have come to call retention metrics or churn metrics, but everybody was so focused on, “Let’s get more customers,” instead of, “Let’s keep them and let’s understand why they leave. Let’s optimize for the customers that come and stay and are profitable for us.”
In this case, the marketing department per se are not even focused on acquisition because it was some the more territory people bringing value to the brand. It was also a very siloed organization. Long time ago, it was also a different market. The US, in that case, in metrics, it’s been more advanced of being fast at adopting metrics and having more consultants and people helping them with the business. There it was isolated in the business of the marketing department was not even thinking about that at all. The fact that they had to think about keeping the money and they didn’t even have the metrics, I thought that there would be something easy, at least, to help or to do and learn to do that.
Then you turned down their offer to go get your PhD. You chose marketing as opposed to math or something more that maybe your professors might have thought you’d go into at that point. What was your intention when you started your PhD? What did you want to study, and what was fascinating to you at that time?
At this point, it was 2 or 3 or something like that when I started applying. This is the era, at least in Spain, of data mining. Now they will call it AI. It was data mining back then. It’s like some companies that have good billing records, now they have data, and let’s celebrate its data. I was fascinated by that. I’m fascinated by the idea that data could be informative of decisions, but I didn’t know what business decisions were or what business decisions people made. The true reason why I started a PhD in marketing was because I did have an internship in marketing and thought it was going to be easier to get in. I applied to business schools and marketing because all I wanted at first was to get in and then I will figure out what to do and do my thing.
That’s what I did. I got into London Business School. In the very first semester, I took the marketing class. It was a class about probability models. It’s a class about how to take customers’ transactions and how to mathematically infer what these people are going to do and why they are doing it. It was a course about how, from the data, to learn customer dynamics and how, if you aggregate all of these insights and data, you can make good predictions. That for me was fascinating.
The fact that I could, from transactional data, infer so many things was the merch of the mathematics and the statistics that I had with the business. Since then, I was like, “I want to be in marketing.” I was very random before that. Since that moment, I was more like, “This is exactly what I want to do.” That’s why I pursued a PhD in marketing where I started collaborating with the Royal Opera House in London, analyzing their customer database, and trying to help them retain the patterns. It all involved more data and figuring out what to do with data. I started in 2005, so it was still early days.
You’ve continued down that path in terms of being able to predict what’s going to make someone leave, what’s going to make them stay, or who’s more valuable. In layman’s terms, what are some of the mathematical ways that somebody could make those inferences early on, or where are the right places, if you were talking to a subscription business or even a retailer, that sees the same customers periodically? Where do they start if they are trying to get their arms around who their best customers are or how to predict who is going to churn and who’s worth saving?
I’m not going to go into methods. I see two main problems that data can help us solve. One is the measurement problem, and the other one is the intervention problem. The way about customer management or managing customers in any way is there’s a measurement problem. You need to measure things and understand quantities.
You need to understand behavior and you need to intervene because the only way to manage is to intervene. If you are thinking about measurement, for me, measurement is a methodology to figure out who are the high-value customers. How can I predict customer lifetime value for example? There is a tremendous fantastic job by my former advisor whose name is Bruce Hardie. He’s a long collaborative with Pete Fader. Dan McCarthy and Pete Fader are big names in this area. They have developed a very good methodology to find out and figure out who is a high-value customer.
The first very class that I was talking about is the class that was called Probability Models, and it was the basis of the work that Bruce and Pete were doing together back then. What it means is you assume that people are making transactions in somehow a random way, but there is something systematic. I’m the person that I’m high frequency and low-value items but could be a different margin, and you are the person who is different frequencies. I like this methodology because it’s probabilistic, meaning I allow errors in the data. It doesn’t mean that the data has to be exact on behavior, but by integrating so many customers, I can predict very well what the people like you will do.
These models are not about, “Robbie, you exactly, tomorrow, are going to buy at 7:00 PM.” It’s not about that. These models are about the people with these patterns who are expected to do this and that and they predict very well. For measurement, this approach was developed by these people. CLV models and probability models are very good.
When it comes to intervention, companies need to think more where it’s not about what is going to happen in the future and identifying the type of customer. For me, intervention is more about, “Should I do it now? Should I do it with you or with others? Should I give you these coupons? Should I give you this?” It’s more of a targeting or a personalization intervention.
When I’m thinking about these problems, I don’t want a probability model that is going to predict aggregates in the future. What I want is something that tells me more about you, the individual customer. For that problem, the intervention, I have seen better results when people apply these newer methodologies for machine learning.
There is now causal machine learning that you can see who responds to which interventions. These methodologies are, in my view and experience, better in identifying who to target, whether to do it now or later and more tactical decisions about intervening. I would recommend companies work in this space to do both. First of all, the first step is measurement. The first step is I want to identify who is my high-value customers. If you going to figure out how to keep them, who to send something to, and how to then engage individually, then the other methodology is better to make those decisions.
I love how you broke it down. I think about some of the practitioners that are reading, especially those that are in a smaller company and might not have junior CRMs to do all of this great thinking before they go off to do their doctoral programs because they are so brilliant. For the average practitioner who’s in the trenches, step one is about being able to measure. This group of customers is more valuable than that group of customers. Customers that look like this, who came in through this front door and made this purchase, or who exhibited this behavior, that group seem to do better than these other groups.
The very first level is simple triggers or simple signals to identify who’s high value or low value. The first step is always measurement. To have a good measurement, I wouldn’t start sophisticated at first. You start with the triggers or with the high signals. It’s like the people who bought this and the people who did that. The next level is like, “Now I want to predict for the customer base and I want to go into the future. Then I would build from it.”
I love this, and I’d love an example of a business like if I had a clothing store. What kinds of triggers might I be looking for? What would be something that you’ve worked with?
One that is big is a channel of acquisition. Everybody understands that depending on how you get the customers that are coming from or a promotion they are coming from to the store. However they are coming from, there’s a lot of differential value in whether customers are coming from different channels. It could be physical channels or online channels.
This is one that I have seen a lot of differences. You look at customers who came from this channel and you realize that they have higher or lower value, but the next question is like, “Within this channel of acquisition, how can I separate those who are the ones who will stick with me versus not?” I have seen a lot of value from understanding the first purchase.
It’s a very technical paper, but the essence of the paper is that we developed this methodology. We call it the first impression. For most retailers, for example, the large majority of customers bought once. If you think about it, there is one piece of information that you have for every customer in your customer base, which is the first purchase. Everybody has a first purchase.
What we did in their paper was that, are there products that are very highly indicative for value? You do find that is the case. The company hasn’t made or done that analysis and you go backwards. You have some model for prediction value that you could use any of the methodologies that we mentioned before. It was by looking at like, “Can I find individual products that were predictive of value?”
To clarify and make sure I’m understanding this, that means that if you are going into Nordstrom, people who buy purses as their first purchase are more likely to come back regularly. People who make it all the way deep into the back of the store and buy lingerie are more likely to come back. You can almost do an analysis where you say, “Here are all the customers that bought a purse first, all the customers that bought lingerie first, and all the customers that bought shoes first, and here’s how much they were worth over the course of the year or how many times each of them returned.” It’s very simple.
The thing is you could think about categories because, theoretically, it makes sense. People who buy maybe shoes are willing to spend more so the company will know that they are higher margin maybe. If I buy something smaller, then I’m going to have a higher frequency so I’m going to come soon after. There are reasons why different categories will have different kinds of value, and this is the first order.
The second order that we got into this that we find in that paper is the paper was a beauty retailer. Looking at the basket composition was already predictive of value. Even with items of similar value, you don’t have to go from shoes to a T-shirt. Not even that radical. We found that there were basket compositions that were predictive of higher value.
Looking at the basket composition of your customers is already predictive of value. - @eascarza Share on XWhat was in the good basket?
I cannot tell you exactly what it was in the good basket. What we did in the analysis was the company had a very large number of SKUs. It was very difficult. If you start looking at them one by one, you are not going to find anything. The whole idea of the paper, what we did is we did a methodology that takes all of these SKUs.
It’s very machine-learning magic why in the sense that you take all these SKUs that were in the whole category of products. In machine learning, we put them later in the space where you don’t have an interpretation of what it is but identify the clusters. Now, what I can do later is that you give me a basket and I can tell you exactly the rating on those clusters and therefore the CLV. The idea was you tell me what you bought, you show me the basket, and I can predict your CLV.
I want to caveat that you don’t predict exactly the CLV. It’s impossible, but it was very good to separate the high-value customers versus the low-value customers. The one thing that we did find that wouldn’t surprise anyone was that when people were purchasing in holidays, for example, Christmas and so on. These people are lower value because many people would come to buy one gift and then come back. There was seasonality.
We are going to look at baskets also depending on when people bought it first. The idea was, “How can I identify the dimensions of the first purchase that are highly lower predictive?” For sure personalities because of the discount. Conditional of that, they were basket compositions that could have a seal where that could be 2X and 3X. It’s so much different.
I love this. I’m sad that you can’t tell us what was in the basket, but I understand. I’m thinking about other businesses I have worked with and I’m thinking about seasonality. Other examples of seasonality are people that sign up for anything relating to managing your financial picture or personal finances. If you come in right before tax season, there’s a pretty good chance that you are going to be out of there right after tax season.
If you come in January as more of a, “This is the year that I get things under control,” you are more likely to stay. If you come in at some random time like July, that means that you are probably pretty motivated. It was counterintuitive that the big spikes of new customers get great volume and have great numbers in that month, but retention and lifetime value would be much lower.
What’s so interesting about that, and this is I think where you are going, is that once you know those facts, then you say, “What do we do with that?” That’s where the interventions come in and you say, “Does that mean that we don’t spend a lot of money to acquire people over tax time even though a lot of people come in?”
Does it mean that we invest more in onboarding those people? My favorite example is you came to Disney+ for Hamilton, which everybody did. We are going to get you to stay for the princesses and the National Geographic documentaries and the ESPN sports matches by surfacing that to you. We have this moment. We know you only came for Hamilton, but we are going to do our darnedest to keep you.
I cannot agree more. This reminds me of one organization I work with that was a year subscription-based. I recommend to, “Let’s do the analysis-based seasonality.” Let’s understand the seasonality in this pattern. Exactly as the example you put with the financial service, what we find is that subscriptions that happen around Christmas were people with higher churn rates the year later.
The company and the people in that team, the first time they saw the data, they were like, “Easy. What we are going to do is we are going to extend the membership not for 12 months but for 13 months because now it’s going to be January.” I’m like, “You are not realizing that these people don’t renew because they don’t see any value. They got this membership. They gift it to someone. Now it was gifted by somebody else. You are missing the point here of the seasonality to understand, ‘How can I provide value for you at a later stage so that you continue?’” I relate so much to that.
It’s funny because something that I admire about you is you are very quantitatively rigorous. You are a sharp thinker. Also, you have that human side and common sense and street sense logic that says, “Let’s think about why this is happening. Why is everybody who signs up at Christmas canceling at the first period at the end of the first year? What could be the reasons? Let’s develop some hypotheses based on intuition. We have this data, what could be the reasons? Which reasons can we test?”
If the answer is they never intended to stay, and they said, “This is the right price point. I’m going to buy these people a one-year subscription,” and the person they bought it from never asked for it and doesn’t want it, neither person is motivated to continue. It’s helpful to know that. It’s also helpful to know that some customers are more valuable than others, and there I have said it. It’s hard to admit, but once you admit it, then you can start thinking about your interventions.
I like what you said about common sense. I love numbers and methods, but the first principle is always common sense. It’s the one that takes you almost all the way there. The more we put methods and data first, the more we lose common sense because we don’t see the forest from the trees. I agree with you entirely.
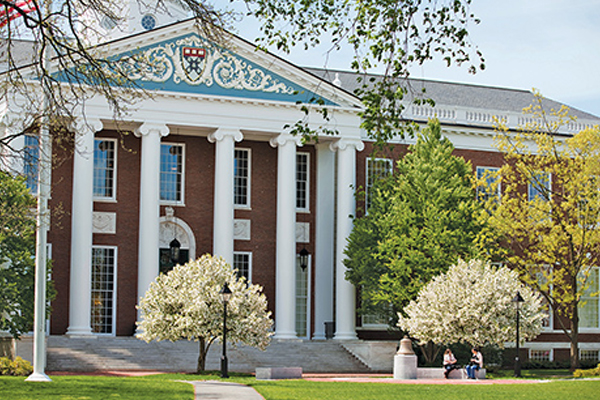
Customer Retention: The first principle is always common sense. It’s the one that takes you almost all the way there. The more we put methods and data first, the more we lose common sense because we don’t see the forest from the trees.
Another thing that I observe is that once you start seeing metrics, you see that seasonality. You see that people are not renewing. There is an obsession with trying to keep them all, an obsession to, “Now that I see these numbers, all I’m going to think is about how to change the number.” Increasing retention is a fantastic thing for the growth of the business, but maybe there are many things that you cannot do and you shouldn’t be doing because you are going to be spending resources on something that has no change.
It is a combination of understanding the numbers but also using your common sense and saying A) What do I think is going on here and what can I adjust? B) Back to the quantitative side, how can I test and see if that is true before I go too far down that path and invest because January is a month for retention? The other thing I thought you were going to say when you are saying extend it to January is a lot of companies I have worked with, if people aren’t renewing, they immediately go to, “Let’s drop the price.”
It is terrible. I’m not saying that every discount is a bad idea at all. I teach marketing. I would be fired if I say so. There are many promotions that destroy value literally. They give the promotion to the person who was going to buy anyway and they destroy value trying to convince the person who’s going to leave anyway. It is true that, for some people, maybe you didn’t find the right price point at the beginning, and changing price is so important to get there. It’s a lever that is so easy to change, but it could be very value-destroying, I believe.
If you promote to people who will buy anyway, you are only destroying value trying to convince them. - @eascarza Share on XHow would I know if I’m running a subscription business and people are canceling at the end of the first period? How would I know if it’s because it’s too expensive, the price is wrong, they tried the product and didn’t like it, or they were never that committed to the product to start with? Either they wanted to watch Hamilton and pay their taxes, or they said, “I’m going to check it out but I’m not sure it’s right for me.”
Two things. One would be with understanding their behavior, especially now that you have a view of what these people are using. In the case of TV, I see what they are seeing. I see that if you were watching every week and the other is saying you unsubscribe, I don’t think that yesterday you were not giving any value. Maybe the price point isn’t good for you.
One of the understanding parts is that I need to understand where your behavior is coming from. By looking at what you do, I’m going to try to get a sense of the value that you get from my service. The other aspect is more interventional. Earlier on especially, I always encourage firms to test in small batches, but I don’t see any harm in testing small promotions because that tells you a lot.
I wouldn’t test promotions only on people that I have identified that will leave me. I will test promotions across the board to see if I’m matching the price point for most of my people or the most population. Let’s say you launched something and you have a 50% churn. It’s like, “I cannot sustain it.” This is all hypothetical.
What you do is you are going to run a promotion. Do not drop the price across the board. Run a promotion from the mindset and look at who is responsive to that promotion. The person who responds to a 10% off is getting value from you because, for 10% off, they are willing to stay. You then compare and see who is more responsive to a price change which helps you understand the proportion of people who would have stayed. Was it 90% of the price for example? It’s a good way to both segment customers for whether they are getting value or not, and second of all, to say like, “Is it that my price pump could be slightly higher?” You then run the scenario, “I would have saved all these types of people.”
That’s interesting because you are looking at both customers likely to stay, and unlikely to stay, and you are looking at with the promotion and without the promotions. You have four different groups. You are learning a lot and probably have a lot of hypotheses about what you think is going to happen, and then you see what happens and you can adjust your understanding. I feel like a lot of businesses don’t understand at a core level why things are happening.
I totally agree with you. If you look at before running an experiment, if you look at your data, and everybody indeed watches Hamilton and nothing else, that’s already telling you that you don’t need experimentation for that. You were deeper into how people are using your service. If you have a good chunk of people who are watching until the very end and then they will cancel, chances are that a better price point will work for them.
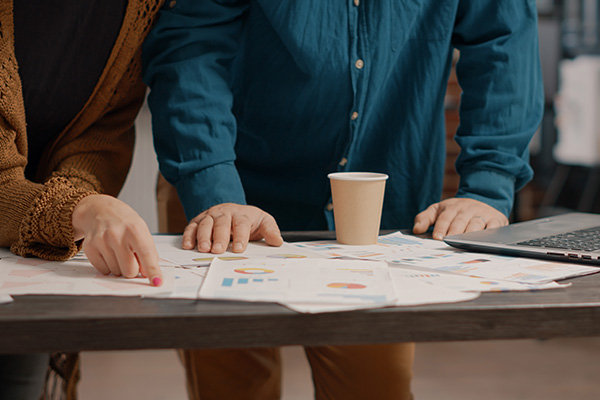
Customer Retention: If you have a good chunk of people watching your videos until the very end and then they cancel, chances are that are a better price point will work for them.
It’s because they are getting value.
I don’t mean to say, “I’m going to analyze the data. I’m going to see everybody who’s watching things until yesterday. Right away, I’m going to send the promotion to all of them.” No. That is what we’ll need to test.
The people who are current who’ve been using the product all along and the person who used it on day one and hasn’t been since they came, you want to see both of them. “Can I get another chance with group one? Does it work to keep group two a little longer or to keep 85% of group two instead of 75% of group two?” If you were managing churn for a subscription business, what would be the metrics that would be most important on your measure dashboard on what you would want to keep track of?
I’m a big fan of dynamics. I believe everything is customer dynamics. The two things that I would have for sure are a pattern or a line. Not a number. I don’t start with a number. I start with a line. I start with a figure that gives me a line over time. It’s way more informative to look at all the cohorts of customers acquiring in a certain period of time and how they evolve over time.
I’m a big fan of what is called cohort analysis. If you come and tell me, “My retention rate is 72%,” it tells me nothing. I have no idea what is a good or bad number for you. If I see the pattern of retention rates over time by cohorts of customers, I can see, “If you have now a drop. It’s your problem. Is there a competition?”
I like the dynamics. I like to see lines over time of how behaviors change. The behaviors I would plot would have usage patterns like ARPU, depending on the service and retention rates over time. Not across the board but across customers who have been acquired at a similar period in time. That’s the only way I can know if it’s our company’s problem or is outside company’s problem.
The cohort could be what month they were acquired in. It could be how long they have been a member. It could be what the promotion was that brought them in or what the channel was that brought them in.
I always start with dynamics on the age of the customer, either cohort that when you were acquired, which is the same as you think, “How well have you been with us?” I believe especially in the context of retention that there is a lot of attrition that is natural. There is a lot of attrition that will always be there. If you plug the line where you take a cohort of customers and plug retention rates, there is a pattern that comes over and over again. By looking at a retention rate across the board, you are hiding these differences. That’s why, for me, I would look at retention rates by people who have been X time with the company and X plus 1, plus 2, etc. I like to see a customer in a natural lifetime. That is what happens along it.
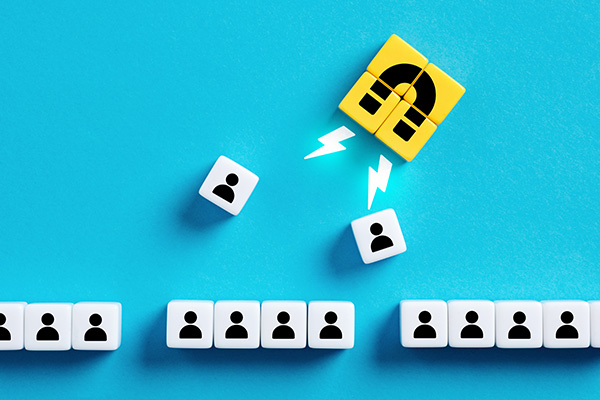
Customer Retention: If you take a cohort of customers and plug retention rates, there is a pattern that comes over and over again. By looking at retention rates across the board, you are hiding these differences.
That would be one thing I would look at, overall patterns of usage and retention over time. That’s the first. The second one would be now more about groups. Now, I hypothesize what would be the triggers for retention. For example, I work with many telecommunications for example. There are triggers that they know predict churn very quickly, which is whether you call customer service on certain things, or whether the competition sometimes launched something X. I would like to see in my dashboard some way to visualize this trigger and how big the trigger behaviors happen. “Which proportion of my customers have engaged in this trigger, this trigger, and this trigger?”
For example, if you had a telco and you know that one of the competitors offered a half-off or some big deal, you’d almost want to note that on those lines that you were talking about and see how big an impact that was and what drove it. You then can design for the next time.
With the triggers also, I designed for the next time, but with the triggers, I then go to this. Let’s say I’m at telco T-Mobile, and then Verizon launched this humongous campaign. We all know that and they know that. What I’m going to do is I’m going to look at the three triggers of behavior. One is a call to the customer service. The other one is a family plan.
Sometimes it’s you have zero usage for 2 or 3 weeks. Imagine these are the three triggers I decided on. Every single week, I keep track of the proportion of my customers that engage this trigger. If Verizon has this new campaign, the first thing I’m going to look at is whether these triggers now happen to way more of my customers, and then I can quickly say, “Tell me the customers who haven’t done this.”
Now you start narrowing the problem that you want to tackle because, at this point, the problem is getting these people not to go there and you know the problem you are trying to tackle. If you give me a number of retention and you say, “I want to increase it,” this is not even a problem to tackle. It’s what I’m going to be doing, which is lowering the price so people increase retention. I want to be more concrete. The dashboard would be important to help the evolution of the business as well as highlight things to tackle when the problems get precise.
If all you have is your retention number and your retention was at 78% and now it’s at 70%, and leadership says go fix it, you as the person responsible for fixing it are going to be much more effective if you know which trigger drove it, and so you can figure out which intervention is appropriate. You can also say, “Which trigger drove it, and do I care? Are these people that I want to invest in? Are these customers that are worth them?” As we said, some customers are more valuable than others. It may be okay with you that Verizon is taking away your least valuable, most price-sensitive, and most disloyal customers or subscribers.
In the case of this Verizon example, I would go and see if the triggers are alarmingly high. I would say, “Who are the customers with these triggers?” I would look at which of those are worth for me to keep and which of those I can do something to keep them.
You are an academic, and you research this. I have two questions for you and I’m trying to think of which order. What is the one thing your students would say about you in terms of what they learned? I’m many years out of business school now, and there are certain professors where there’s one thing that I still remember that they taught me and it’s still there front of mind. What is it that you are hoping that they took away that they didn’t know before they were there?
It’s a tough question. I teach two courses. One thing that they say, and I hope they say, “It’s not about the content. It’s about the execution.” They say that I push back a lot, and I want to make them think. Students come to class and leave the class and like, “Now I think more about these problems. I think differently. I think better.”
I always insist on the thinking because if you don’t think first, your numbers are going to say or whatever your data scientist is going to say. You are not going to help the business. I hope that they would say that the professor make us think. That’s one of them. The second one is more in the context of the conversation we are having. One of the things that I always try to insist on is that when it comes to customer retention, many students come to say, “Let me identify the customers who are likely to churn.”
I have a few classes on that. In the class, they hope that they leave the class thinking, “First of all, I am going to think about why they are churning and what intervention I could have to change that behavior.” This is very in the context of this marketing problem. It relates to the other one. You have to think. I hope you think about why the behavior is this and how the data analysis will help you there. If you don’t think, you are going to be blurred.
This is so important because it is easy to get overwhelmed by the data and to look at the data and then say something, but it is to step back and say, “What is this data trying to tell me first of all?” Measurement. What do I understand? What have I learned? What are the implications? What are my options? What are my guesses as to why this is happening and how do I figure this out?
Like you said, it requires common sense. It requires thinking. It requires pushing yourself or your team members to say, “What could be causing this weird thing with the data?” A couple of questions and then I’m going to wrap it up. I’m interested in what’s next for you and what you are studying now. You are the Cofounder of the Customer Intelligence Lab at the DQ Institute at Harvard Business School. Your mission is to help organizations use their valuable customer data effectively and responsibly. They seem like they might be at odds effectively and responsibly. What are you trying to do here?
For most of my career, what I have done is develop methods or insights into how to increase the impact of interventions. Increasing retention is one of them, increasing value or whatever. I have been preaching about the value of data because data help us get in there, but two things are at odds now in my mind.
One relates to the more you intervene, the more you personalize, and the more you do what this data-driven or AI approach is telling you, the more risks are there to end up with interventions or marketing tactics that you were not intended to do. For example, you could find yourself giving price promotions to certain parts of the population. You could be preventing some people to access certain products in certain places. In the journey towards precision, efficiency, and effectiveness, we might lose that.
The more you work with data-driven AI approaches, the more risks you are going to end up with and interventions that you did not intend to do. - @eascarza Share on XThat is a big discussion around algorithmic bias and unfair outcomes. I’m not blaming the algorithms only. I’m blaming the people who are using the algorithm. This data-driven marketing has become very precise to the point that sometimes we can exclude customers that we didn’t mean to exclude. That’s one of the aspects of the responsible use of the data. Meaning the outcomes of these data decisions are aligned with your intentions.
If you did not intend to change prices separately by race or gender, then you need to do it. You need to know it. That’s one of the missions of the lab. With my copy, we develop an algorithm that helps you personalize an intervention but always make sure that your outcomes where whatever the company is going to do is equally likely to go to certain people in the population, however you define it.
The other aspect of the responsibility is that there is now the issue of data privacy. It is growing and growing. It’s going to continue growing because we need to protect data and what customers want to be protected in their identities, their privacy, and so on. Every single step towards data privacy, by definition, is at odds with getting value from data.
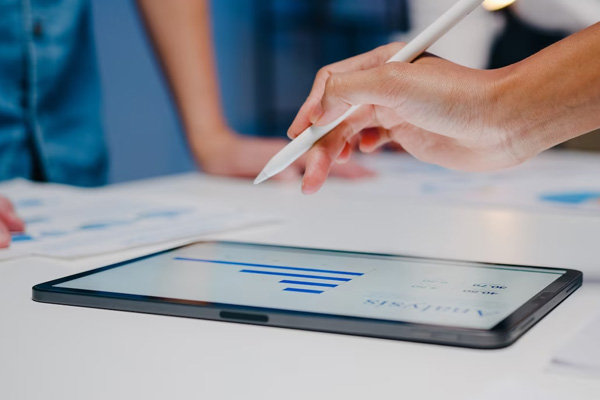
Customer Retention: Every single step towards data privacy is at odds with getting value from data.
There is this efficiency and privacy trade-off. Part of the projects that I’m working on now with some of my students here is given that now data is going to be tackled differently, we are going to collect data differently because there are methods to make the data more private. Can we develop new methods that are as effective as before or as much as you can in effectiveness, knowing that the data is going to be protected for privacy reasons?
As of now, companies are all worrying about privacy because it comes by the law. Many companies are seeing privacy as a legal requirement, but it’s more than that. The more we treat data with more privacy, the less we can do with the data. It’s by definition. We need to think strategically about what the marketing actions and the interventions are that we use data for, and what are the implications for respecting privacy, which is coming, and which we should on how to manage this balance and this part of what we are doing in the lab.
Is this where your students are coming up with rules and principles that they are pushing out onto employers and companies, or are the companies interested in figuring this out?
The lab is mainly in collaboration with companies. What I say to my student, mainly my PhD student, is the idea of the lab is we are collaborating with many companies that we have a brochure with a few surveys so we can screen companies like, “How can we more helpful in both sides?” Some companies are at the level that, “I have a lot of data. I don’t know what to do with it.”
Other companies have been doing more data analysis for a longer time. They already are data-driven, but they think they could do better. Many others have been doing this for a long time, but now they are worried, for example, that they might have some algorithmic bias in their practices. They reach out to us. The way we collaborate with them is that I always want to run an academic paper that everybody can read. It’s not the goal to consult with this company per se. It’s to develop tools and develop frameworks. We can write about as professors that will help everybody else, including this company.
It’s great. It’s so important and I personally am very worried about it. There are not a lot of laws. Laws can’t keep up with what companies are able to do. I’m appreciative that you are doing this.
These are big steps that we need to take in the future. Now, it’s a very big problem, but this is the one we are trying to tackle now.
It’s a good place to start. A lot of tomorrow’s leaders are at Harvard Business School and a lot of the execs that are mid-career doing your executive education programs. I hope they read this and I hope that they take it to heart. Fingers crossed. I could keep talking to you all day, but let’s close out with a little speed round for fun. First subscription you ever had?
I’m going to show how boring I am. Amazon Prime, 2006 or 2007. Something like that.
Your favorite subscription that you recommend to other people.
New York Times. Again, I’m going to show you how boring I am.
Favorite place to hang out in Cambridge.
Waypoint $1 oysters every day before 7:00 PM. I didn’t mean to be promotional that I’m talking about this.
That’s totally fine. It’s great. Most compelling course you’ve ever taken.
The most compelling course that I mentioned is the BNC program. It was not compelling per se because it was very focused, but it was a game-changer for me.
Something you are learning right now.
Differential privacy, which is one of the methods I tell you about to make the data more private.
Eva Ascarza, thank you so much for being a guest on the show. I know my audience is going to learn a lot from you, so I appreciate you taking the time to teach us and to talk to us, so thank you very much.
Thank you so much. That was a lot of fun. Thank you so much for inviting me. That was great.
—
That was Eva Ascarza, Jakurski Family Associate Professor of Business Administration of Business Administration at Harvard Business School. For more about Eva, go to EvaAscarza.com. For more about subscription stories, go to RobbieKellmanBaxter.com/podcast. If you like what you read, please go over to Apple Podcasts or Apple iTunes and leave a review. Mention Eva and this episode if you especially enjoyed it. Reviews are how readers find our show and we appreciate every one of them. Thank you for your support and thanks for reading.
Important Links
- Eva Ascarza
- Harvard Business School
- Customer Intelligence Lab
- Eva Ascarza, Associate Professor, Harvard Business School
- Bruce Hardie, Professor of Marketing, London Business School
- Dan McCarthy, Assistant Professor of Marketing, Emory University
- Pete Fader, Professor of Marketing, Wharton School of the University of Pennsylvania
About Eva Ascarza
Eva Ascarza is the Jakurski Family Associate Professor of Business Administration at the Harvard Business School (HBS) Marketing Unit. She uses tools from statistics, economics, and machine learning to answer relevant marketing questions. Her main research areas are customer management (with special attention to the problem of customer retention), Marketing AI, and algorithmic decision making. She uses field experimentation (e.g., A/B testing) as well as econometric modeling and machine learning tools not only to understand and predict patterns of behavior, but also to optimize the impact of firms’ interventions. Prof. Ascarza is a co-founder of the Customer Intelligence Lab at the D^3 Institute at HBS.
Love the show? Subscribe, rate, review, and share!
Join the Subscription Stories Community today: